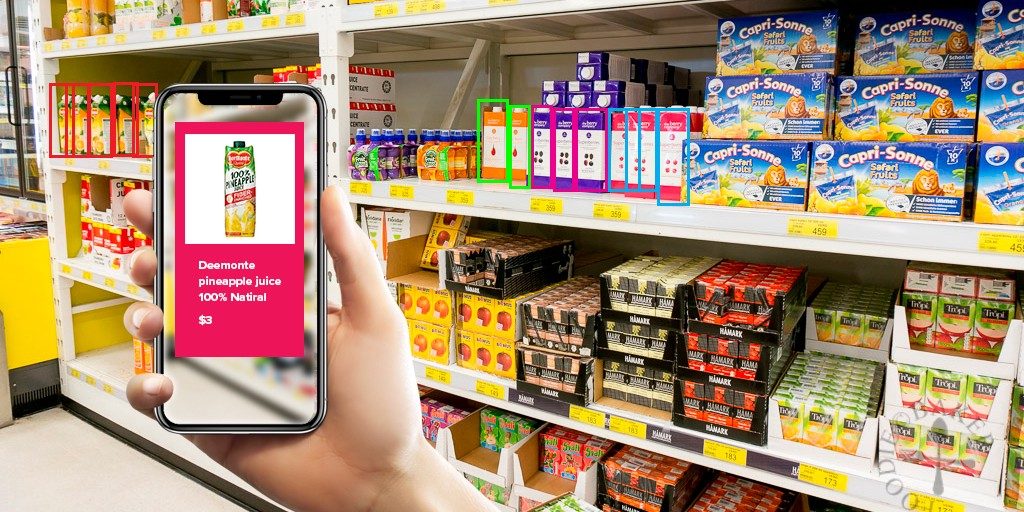
With the e-commerce boom, entrepreneurs have learned that conventional strategies of visual merchandising or sales promotions won’t be able to sustain profits in the cutthroat CPG industry. Many retailers are already implementing AI and image recognition to deliver the next level of customer experience, bringing the dawn of a new era for the retail industry. According to Gartner, by 2020, 85% of customer interactions in the retail industry will be managed by AI. Product discovery, product recommendations & trend analysis are some areas for implementation of computer vision and image recognition. This article elaborates on how image recognition can be implemented by retail & CPG companies.
1. Auditing Product Placement:
Customers are making key buying decisions at store shelves and companies have to use technology to stay ahead of the fierce competition or face extinction. Gathering key consumer information helps companies understand their needs better. Shelf recognition using computer vision digitizes store checks and is important in gathering key consumer information through AI.
Computer vision using deep neural network detects objects within images of shelves and classifies them based on category, brand, and item. This is helpful in streamlining manual, time-consuming and error-prone store audits of SKUs so that the manual effort is directed to the actual task of selling the products.
2. Detecting trends in product placement:
The placement of a product in a store can either make the product sell like a hot cake or stay forever on the shelf. Companies like Tesco have already initiated the use of image recognition software to improve product placement in its stores. Image recognition uses photographs of shelves or hotspots and quickly gives insights into consumer behavior, preferences, and placements of products on display. With AI being able to interpret humungous amounts of data & provide valuable insights, something that is impossible for humans to accomplish with accuracy, strategists can learn the movement of products, how they are consumed or how well product placement works work towards getting maximum ROI from the placements and advertising messages. The AI engines also generate insights on how a brand’s product is being used across social media and accordingly enable marketers in their efforts.
3. Assessing compliance and competition:
Most companies depend on sales reps to audit the company product placements, brand presence and compliance at outlets. This can be a tedious process. Image recognition can be used to track compliance with the merchandising standards for each outlet & have better trade program adaptations. Machine vision is also used to track the competitor brands, their placements, brand presence, and strategies through competitor’s distribution, channels, & shelf strategies and provide important business suggestions. For example: if a product is not performing against a competitor product, it may suggest a different product placement or generate discounts.
4. Category analysis:
When you walk into a supermarket, you are most likely to find a product from P & G, if you are looking for something in beauty and pharmacy categories. This is a result of the company staying vigilant with consumer trends & competitor behavior regarding the product and categories. Using Vision APIs that quickly classify images into categories, identify the stocking of the competitors in that categories, & the competitor offers, enables P&G to devise new marketing scenarios leading to higher shelf share.
In short, image recognition technology is helping retailers and manufacturers understand their marketplace and react in real-time. The trend is moving from online to offline use of image recognition in the CPG industry. Aspects such as compliance or calculation of a product’s performance compared to its competitor can be projected in a better way than with manual processes where there’s a chance of human error and problems in process scalability. And that’s not the end, image recognition is further evolving allowing the consumers to directly engage with the manufacturers via wearable devices. So why not embrace this new trend and automate?